BLOG
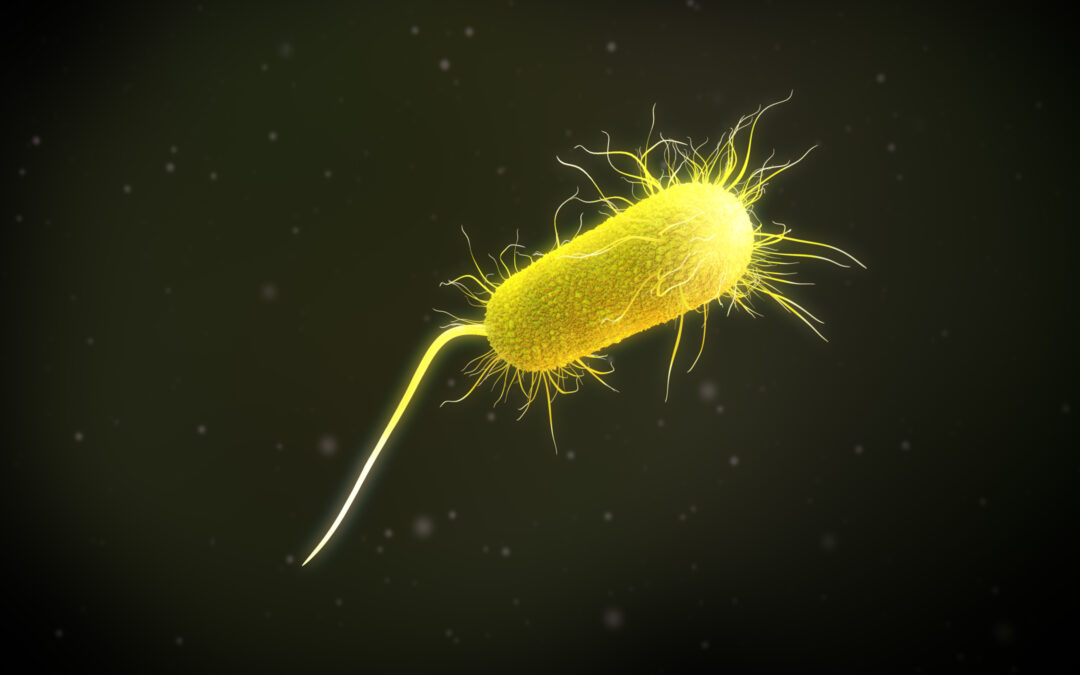
New Compounds with Potent Antibacterial Activity and Desirable In Vitro ADMET Profiles
In this work, we introduce the first multitasking model for quantitative structure-biological effect relationships focused on the simultaneous exploration of antibacterial activity against Gram-negative pathogens and in vitro safety profiles related to absorption, distribution, metabolism, elimination, and toxicity (ADMET). The multitasking model for quantitative structure-biological effect relationships was created from a data set containing 46,229 cases, and it exhibited accuracy higher than 97%
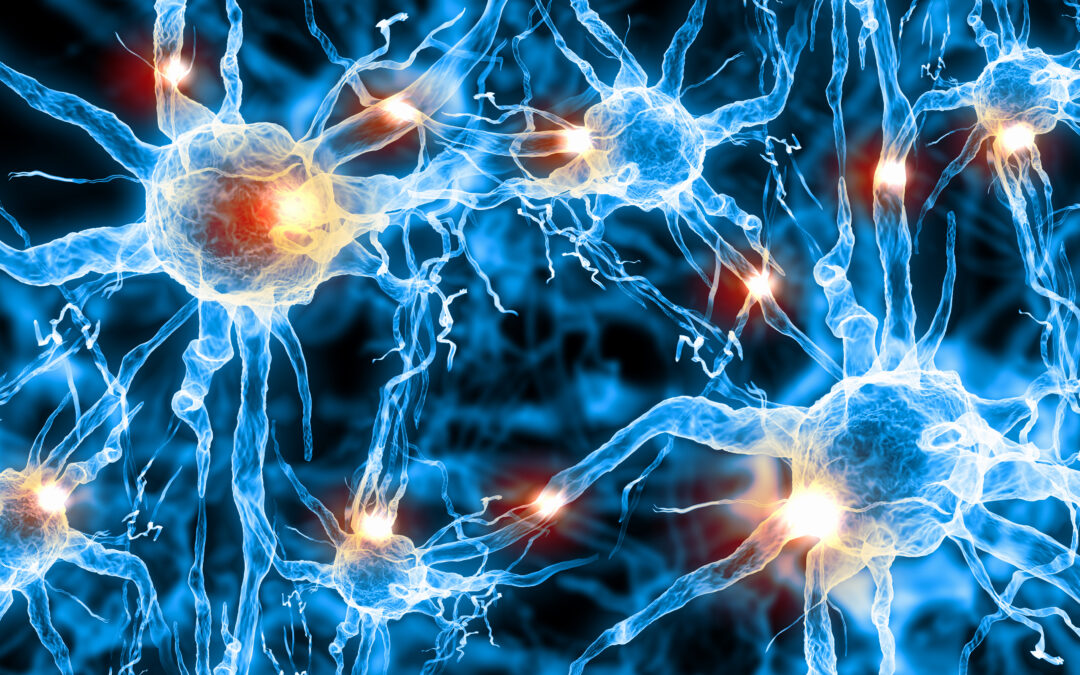
PTML Modeling for Alzheimer’s Disease: Design and Prediction of Virtual Multi-Target Inhibitors of GSK3B, HDAC1, and HDAC6
We assembled ten molecules from several fragments with positive contributions. Seven of these molecules were predicted as triple target inhibitors while the remaining three were predicted as dual-target inhibitors.
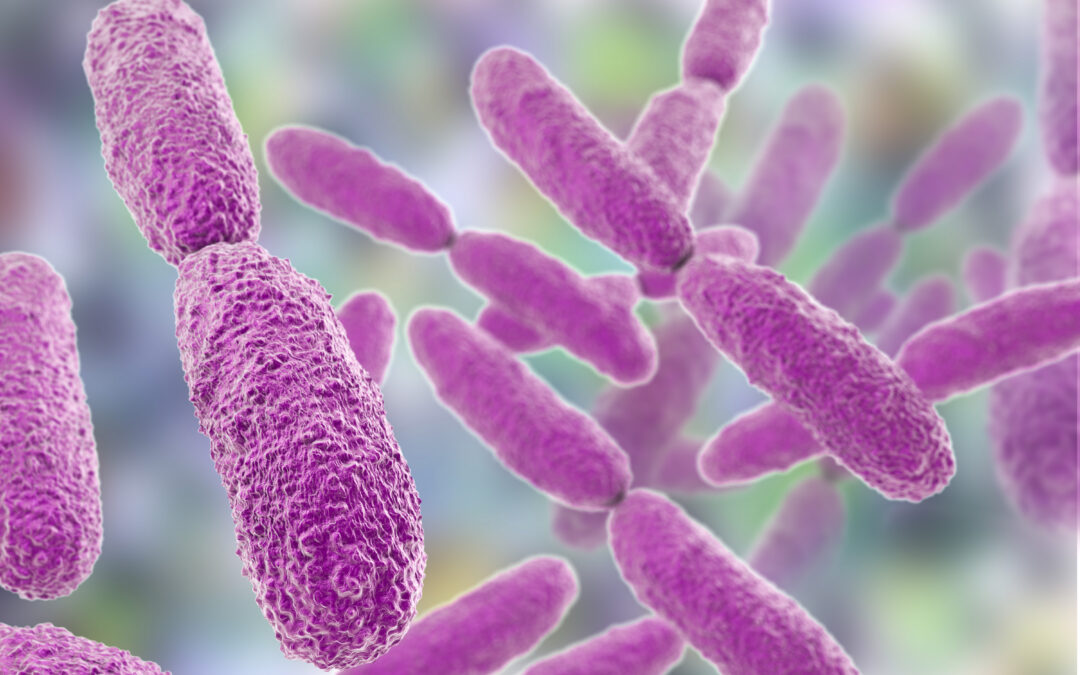
Enabling the Discovery and Virtual Screening of Potent and Safe Antimicrobial Peptides. Simultaneous Prediction of Antibacterial Activity and Cytotoxicity
This work introduces the first multitasking (mtk) computational model focused on performing simultaneous predictions of antibacterial activities, and cytotoxicities of peptides.
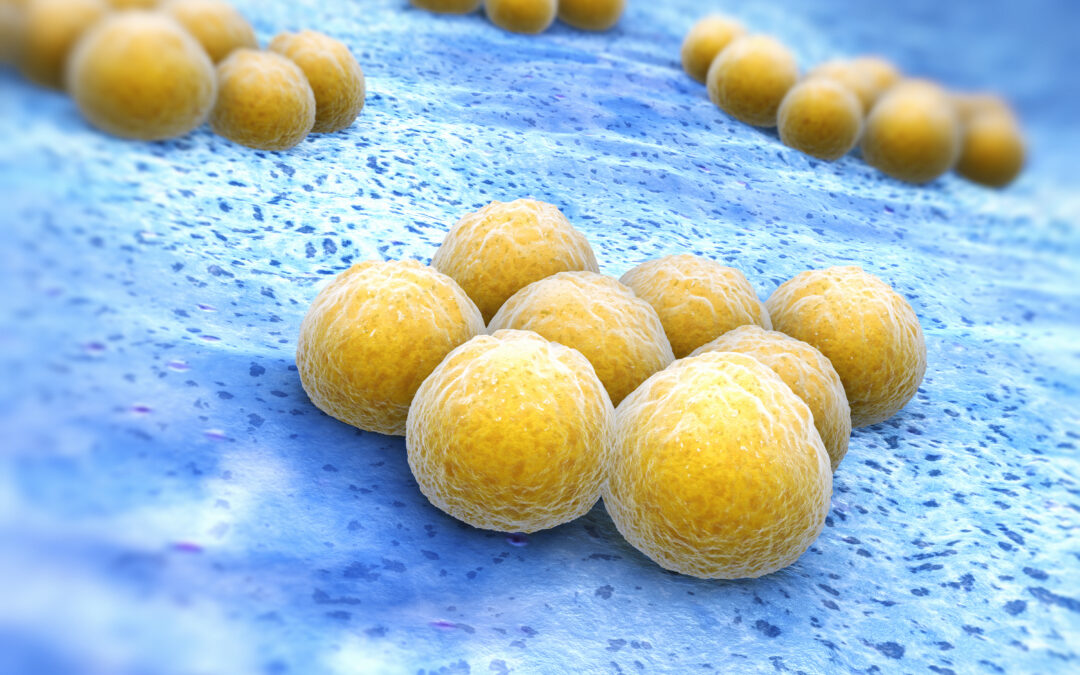
First Multitarget Chemo-Bioinformatic Model to Enable the Discovery of Antibacterial Peptides against Multiple Gram-Positive Pathogens
Here, we introduce the first multitarget (mt) chemo-bioinformatic model devoted to performing alignment-free prediction of antibacterial activity of peptides against multiple Gram-positive bacterial strains.
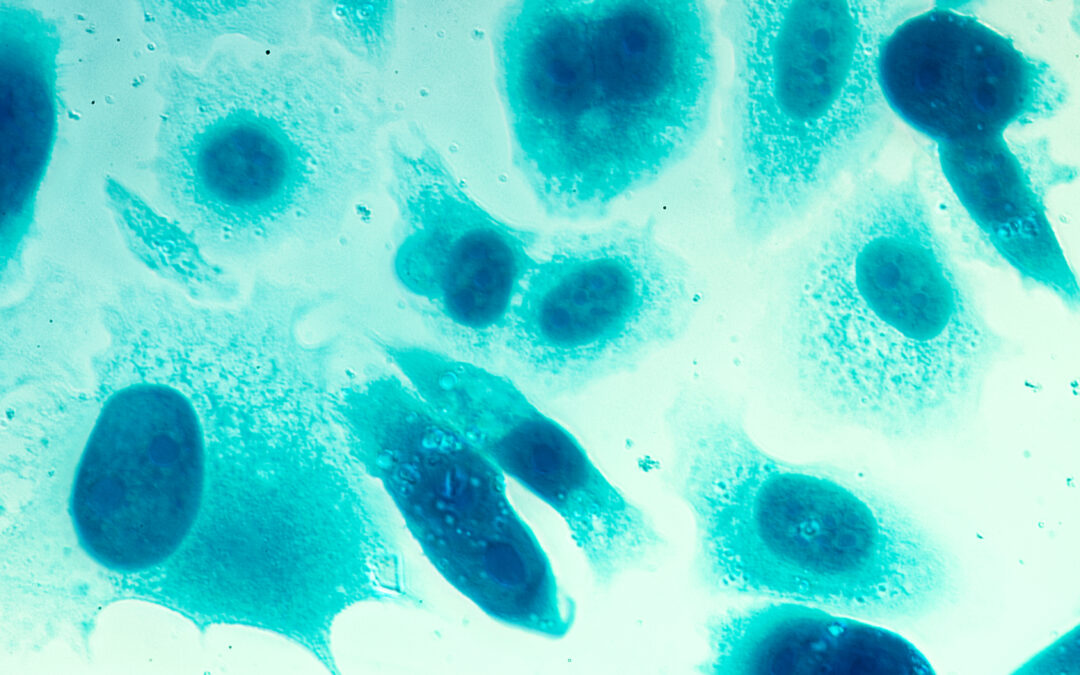
Speeding Up the Virtual Design and Screening of Therapeutic Peptides: Simultaneous Prediction of Anticancer Activity and Cytotoxicity
Here we propose a novel computational methodology for the virtual design and screening of peptides with potential anticancer activity against different cancer cell lines, and low cytotoxicity against diverse healthy mammalian cells.
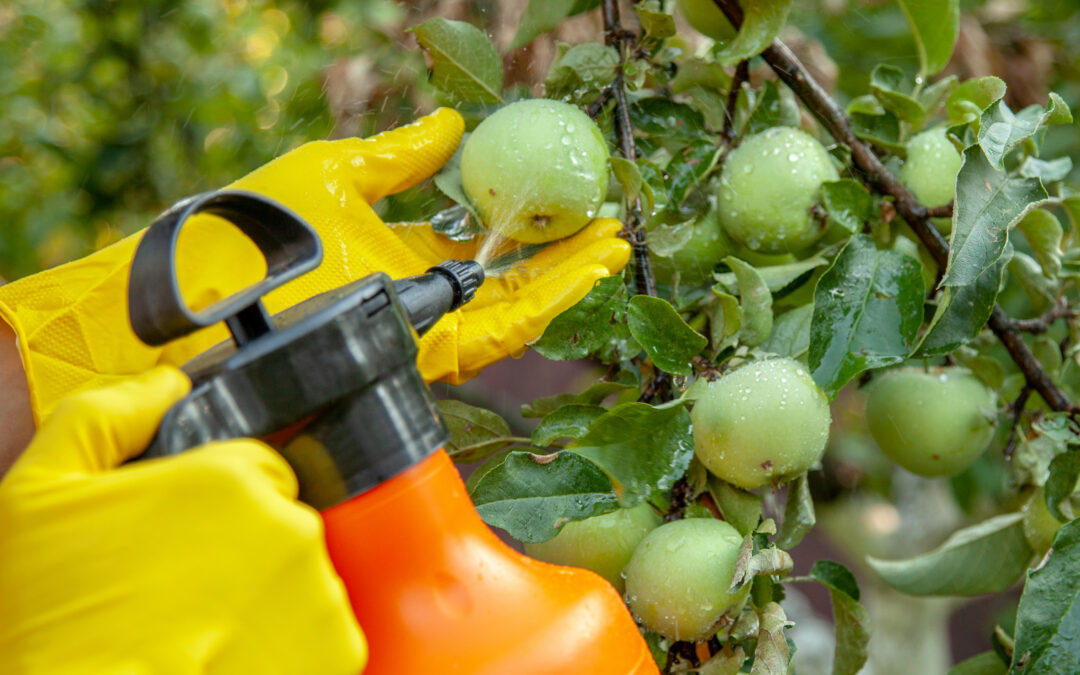
Rational Design of New Agrochemical Fungicides Using Substructural Descriptors
his constitutes an alternative for the discovery of compounds that are able to decrease crop losses caused by phytopathogenic fungal species. The discriminant model based on substructural descriptors provides a promising methodology for the development of molecular patterns to be used in the design of, search for and prediction of agrochemical fungicides of wide spectrum.
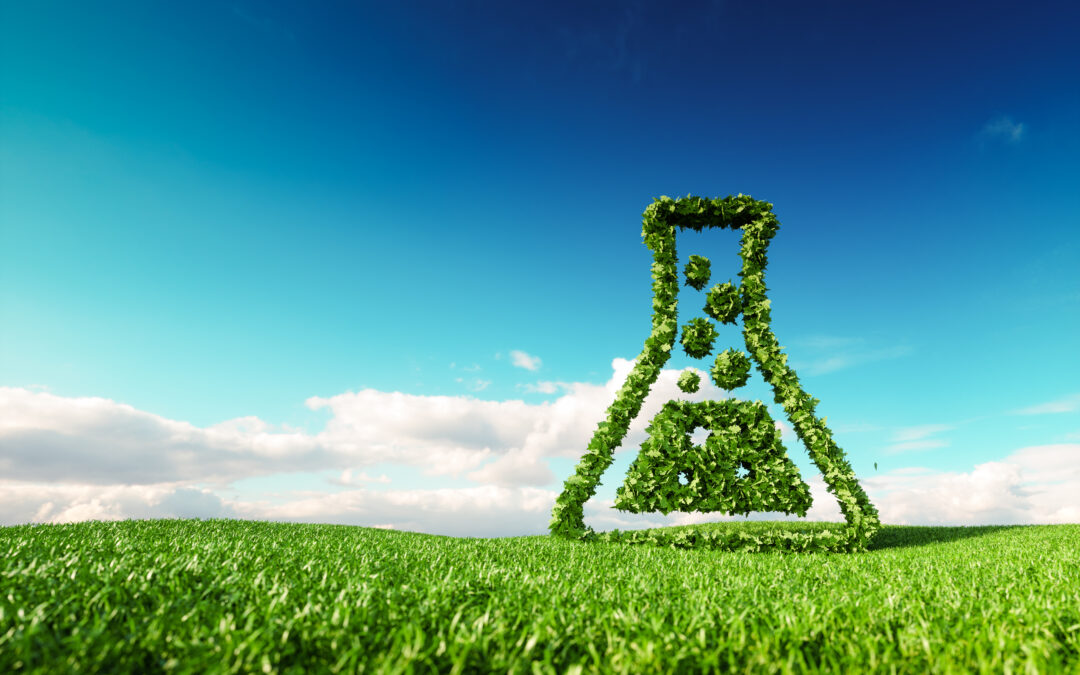
Fragment-Based Approach for the In Silico Discovery of Multi-Target Insecticides
The present study is focused on the development of a fragment-based approach for the in silico discovery of multi-target insecticides from a heterogeneous database of compounds. The present methodology was based on a QSAR discriminant model which classified correctly more than 90% of insecticides and inactive compounds
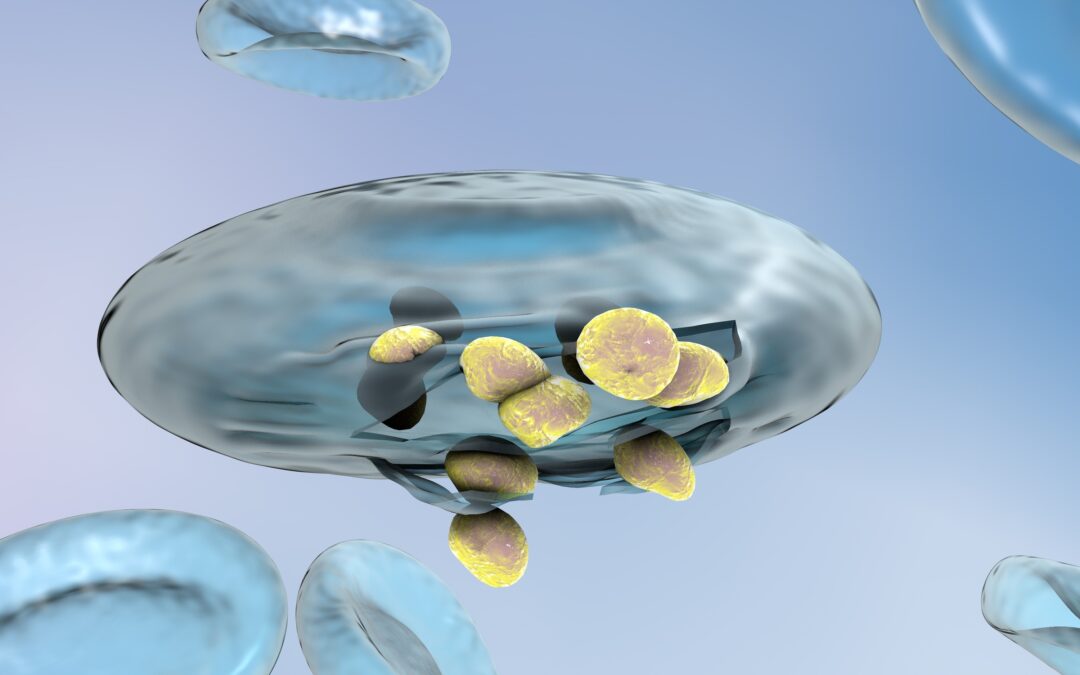
Demystifying Artificial Neural Networks as Generators of New Chemical Knowledge: Antimalarial Drug Discovery as a Case Study
Six of these molecules were predicted by the ms-QSBER-EL model as potent and safe antimalarial agents. The designed molecules complied with Lipinski’s rule of five and its variants.
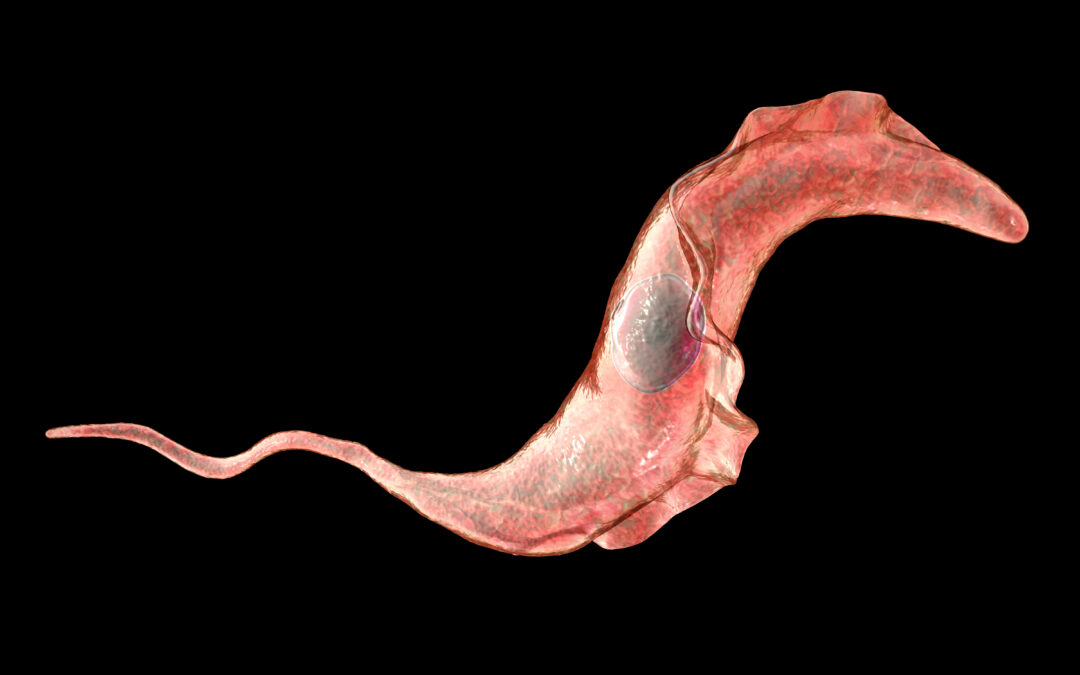
QSAR Modeling for Multi-Target Drug Discovery: Designing Simultaneous Inhibitors of Proteins in Diverse Pathogenic Parasites
Several fragments were directly extracted from the physicochemical and structural interpretations of the molecular descriptors in the mt-QSAR-MLP model. Such interpretations enabled the generation of four molecules that were predicted as multi-target inhibitors against at least three of the five parasitic proteins reported here with two of the molecules being predicted to inhibit all the proteins.
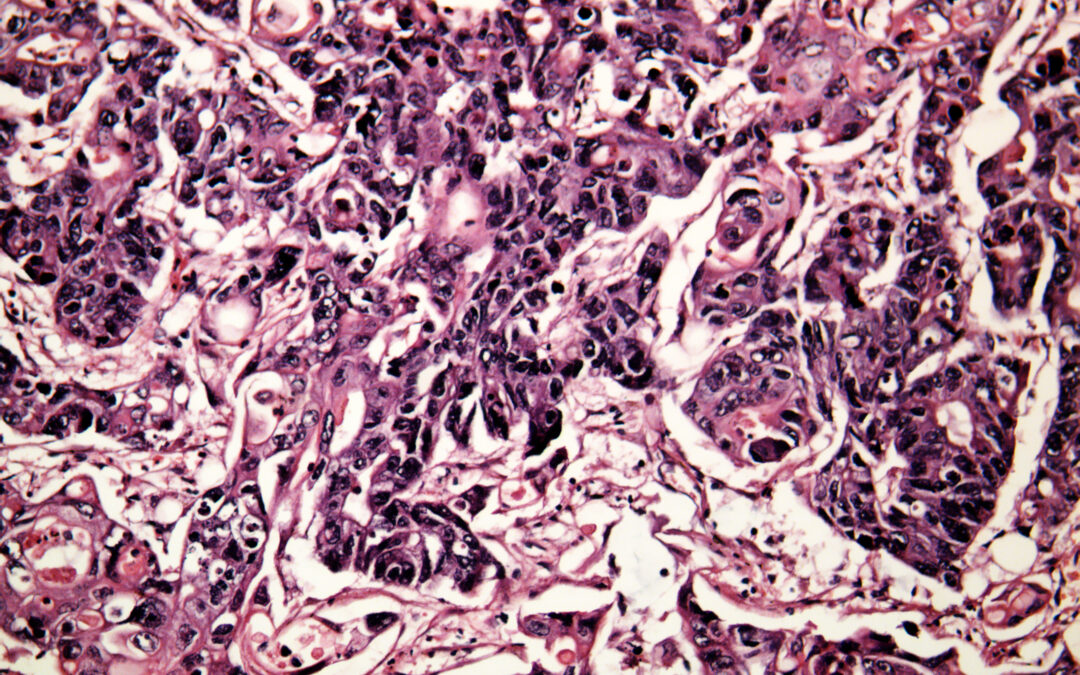
Cell-Based Multi-Target QSAR Model for Design of Virtual Versatile Inhibitors of Liver Cancer Cell Lines
we report the development of the first cell-based multi-target model based on quantitative structure-activity relationships (CBMT-QSAR) for the design and prediction of chemicals as anticancer agents against 17 liver cancer cell lines. While having a good quality and predictive power (accuracy higher than 80%) in the training and test sets.